Only if you make an individual offer to each client, one that they can’t refuse 😉 But this is a hellish task that should be handled by a bunch of employees 🙁 However, this platform has learned to do this without human involvement. That’s already cool. But the main thing is that the recipe for its creation can be used to develop in-demand platforms in other areas too.
The Essence of the Project
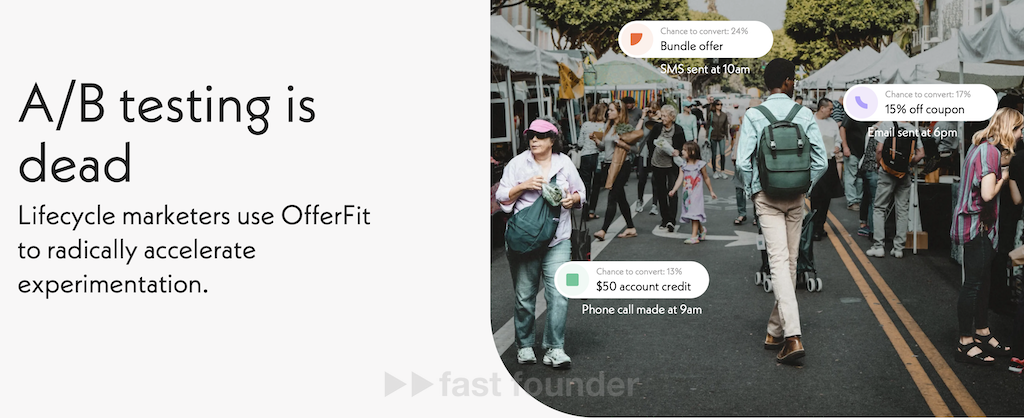
OfferFit claims that “A/B testing is dead”—at least in the realm of sending marketing offers to business clients.
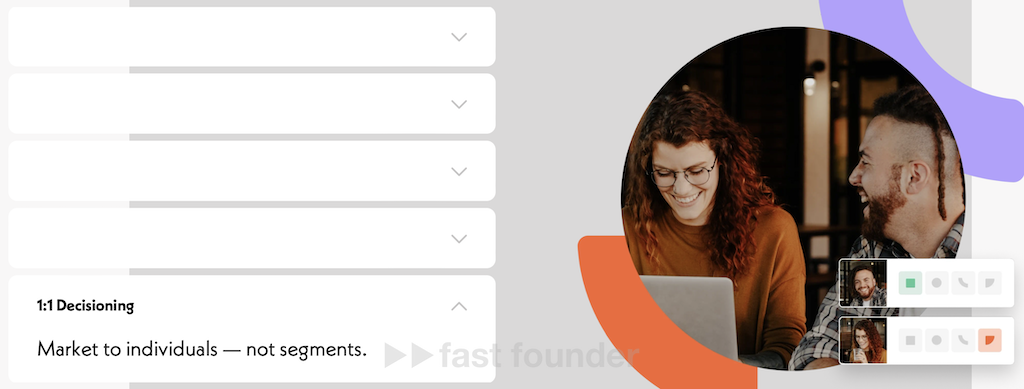
A/B testing is commonly used to find the most effective marketing offer for a particular segment of the customer audience. And it has two natural drawbacks:
- It searches for an offer that shows optimal average results across the entire customer segment.
- Once the optimal offer is found, it continues to be used until it stops working altogether.
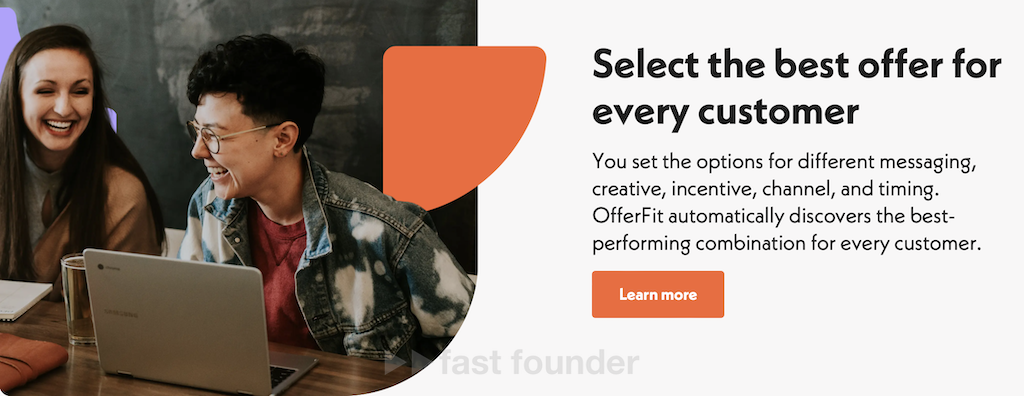
OfferFit proposes to make effective offers to each customer individually—choosing the most suitable formulation, creative, incentive to purchase, channel, and timing of the offer delivery for them.
Furthermore, the combination of parameters found for the client is not frozen forever. If the next offer made to a client, based on a once-prescribed recipe, stops showing previous results, the platform automatically starts searching for new, more winning combinations of parameters.
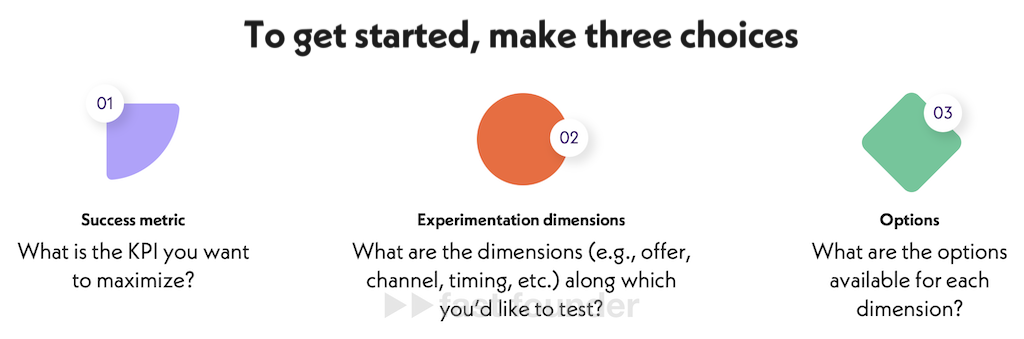
To get the platform started, you need to tell it what business metric you want to optimize (conversion from offer to website visit, conversion to purchase, order size, etc.) and which offer parameters can be changed (formulation, creative, channel, or delivery time, etc.). You also need to define the possible options and/or limits for changing each parameter (range of discounts, list of current promotions, marketing channels used, etc.).
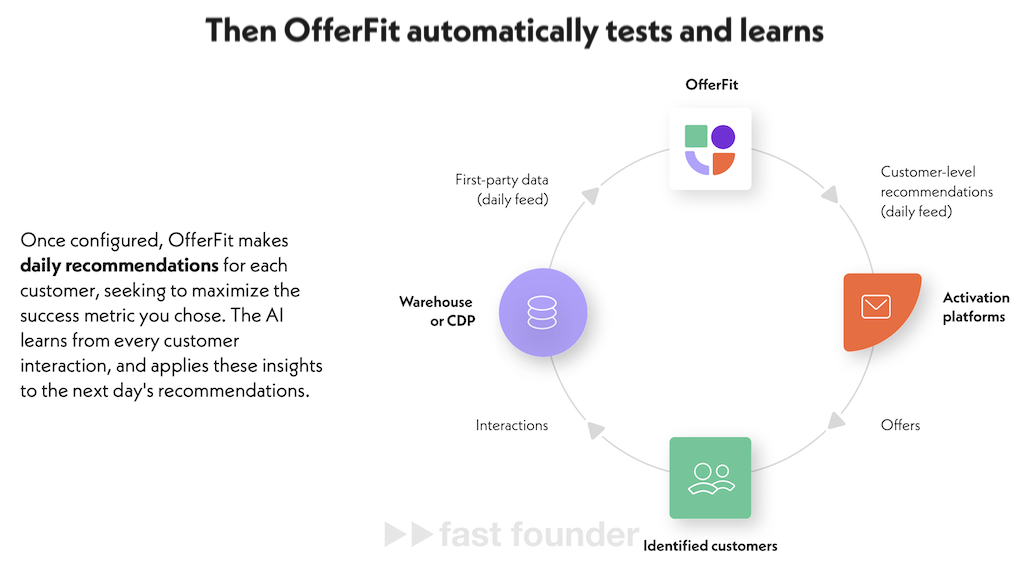
After this, OfferFit independently and automatically conducts daily experiments with offer mailings, trying to maximize the target metric.
Every day, the platform gets new results and applies the knowledge to the experiments it will conduct tomorrow. Thus, it constantly improves and adjusts to possible changes in customer preferences and habits.
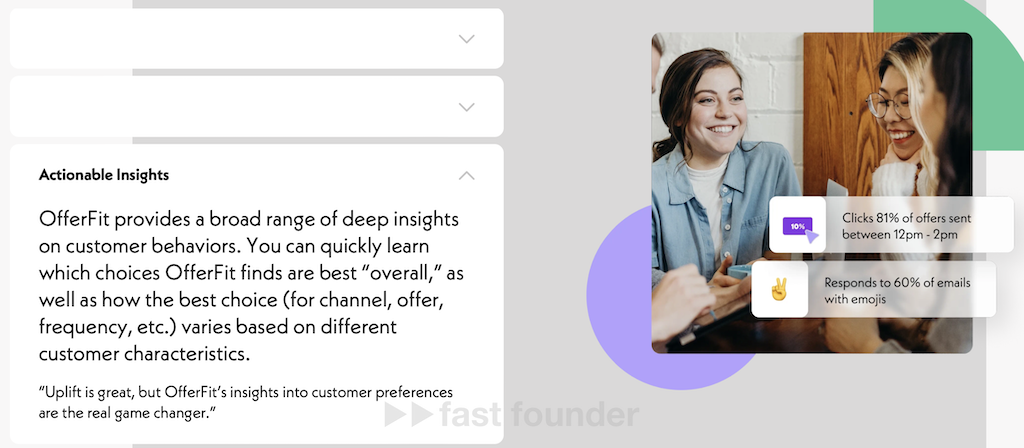
A daily updated list of insights across the entire database and for each client can be seen on a special dashboard. For example, you can find out that Vasya Pupkin clicks on offers sent to him via SMS between 12 and 2 pm, while Masha Ivanova only responds to offers with discounts of no less than 20%.
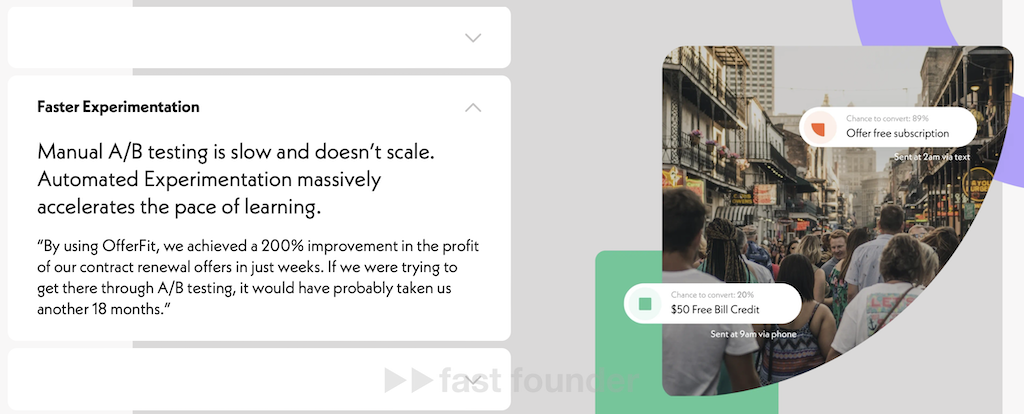
Automatic experimentation critically improves the time to achieve optimal results. One client says that with the platform’s help, they tripled the number of contract renewals in just a few weeks, while similar results through manual A/B testing would take no less than 18 months.
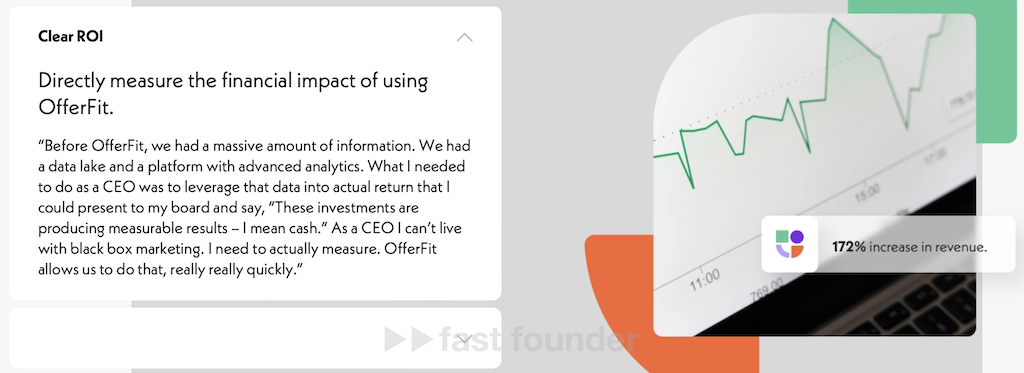
OfferFit reports daily to management on the key performance indicators achieved the previous day. This serves as a daily reminder of the important work it performs and the contribution it makes to the business—so the company doesn’t forget to renew its subscription to the platform 😉
The subscription cost is not explicitly stated on the website, but it surely depends on the size of the customer base and the number of offers sent per month.

The platform is genuinely effective, with clients including the well-known gaming company Wargaming.
OfferFit has now raised $25 million in new investments, thereby increasing the total investment in the project to $39 million.
What’s Interesting
The key phrase is in the client’s review mentioned above: “We could have achieved the same results manually, but only in 18 months instead of a few weeks.”
In other words, OfferFit doesn’t do anything extraordinary. Their platform accelerates and cheapens what a company or person could otherwise do.
This is the common recipe for most modern AI platforms. And it’s an effective recipe—customers eagerly buy such platforms, and investors invest in them. Here are just a few recent examples.
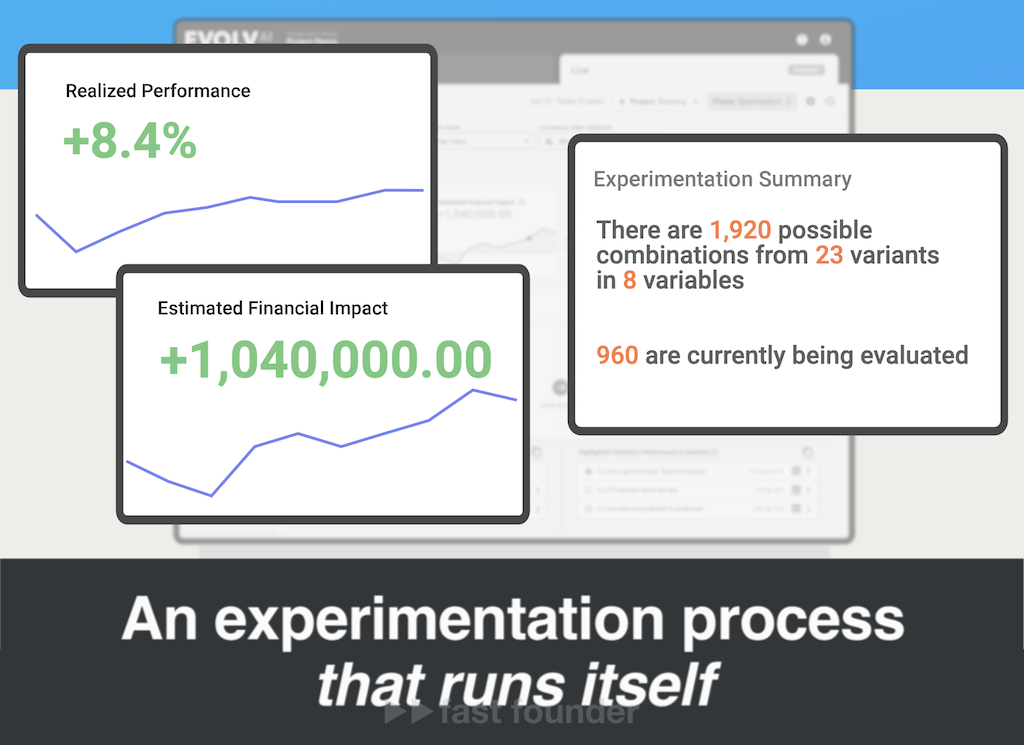
Evolv AI, which I wrote about a few days ago, made a platform for improving website designs. It takes key design elements and automatically tries different combinations (font size, color, placement, images) to achieve maximum target metrics—conversion to purchase, order size, time spent on the site, etc. They raised $23.3 million in investments.
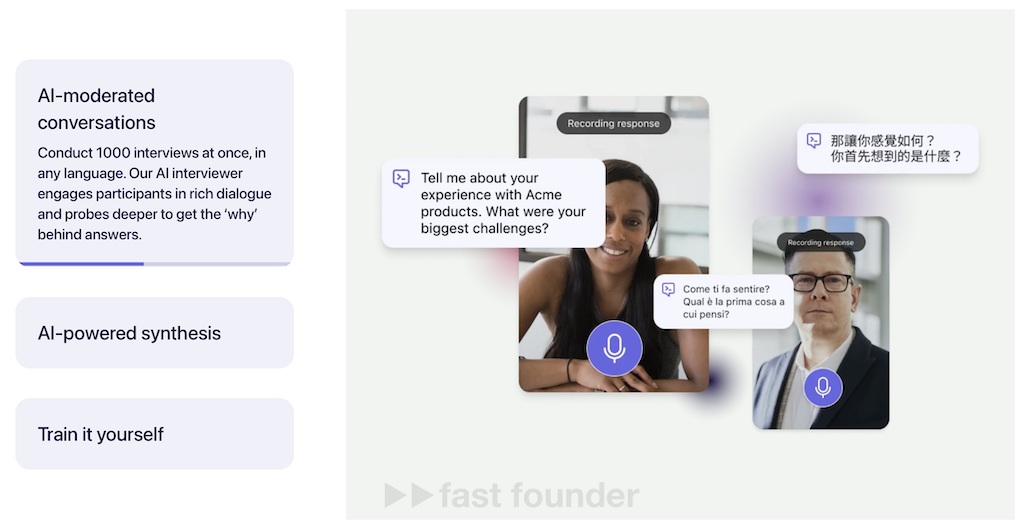
Outset, which I also wrote about recently, made a platform for conducting marketing interviews with consumers. The platform’s AI machine conducts the interviews itself, striving to get substantive responses from users, then analyzes the responses and generates a comprehensive report on the conducted research. They raised $4.9 million in investments.
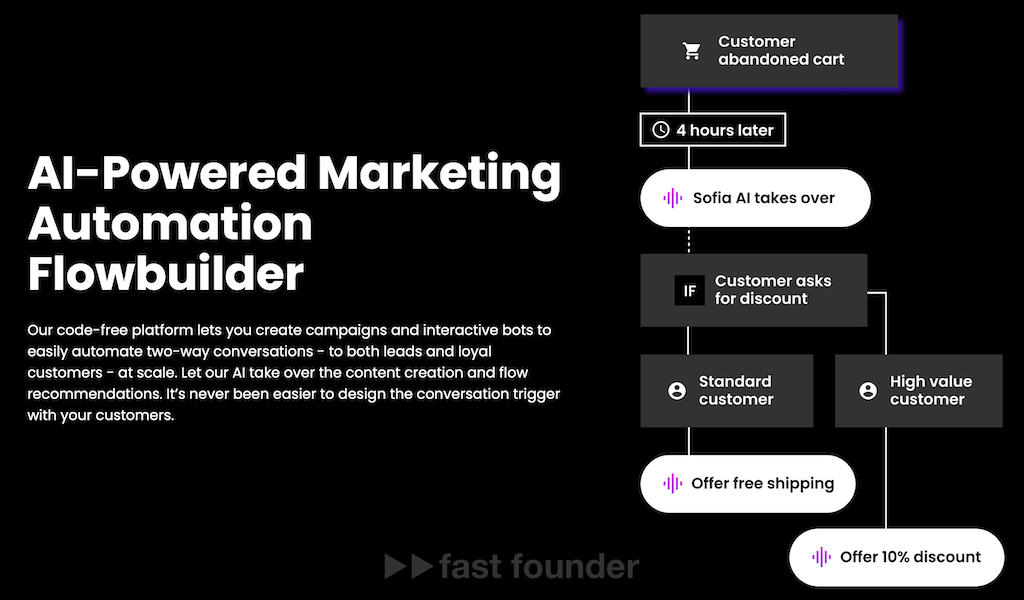
Connectly, which I wrote about a couple of weeks ago, made an AI salesperson for online stores that automatically connects with customers who abandoned their carts on the website and tries to lead them to purchase—offering discounts or similar but more suitable products. They raised $17.25 million in investments.
All the above actions can be done manually, but this would take a lot of time, require the involvement of many live employees, and ultimately lead to additional expenses and lost profit due to unutilized opportunities.
Where to Run
If there are employees in a company’s back office, you can bet they’re engaged in manual work 😉 It’s hard to imagine an office where employees only think about what else useful they can do for the company.
This means there are a huge number of yet untapped opportunities for implementing AI platforms within the scope of the recipe described in the review. You just need to see what people don’t have the time or quality to do manually and shift that work onto the shoulders of AI.
This is the general direction of movement—creating AI platforms capable of automatically doing the same things that people could do manually but don’t do with the necessary frequency and/or detail. Just because it would take too much time and require a large number of employees.
Because of this, for example, companies optimize sending offers not for each client, but for whole client segments, and repeat the re-optimization process quite rarely—although it is theoretically possible to do it manually. And OfferFit took it upon itself to perform this procedure with the necessary detail and daily.
What are company employees doing now—but rarely? Would the company’s work results improve if employees did the same thing but much more often? Can this work be automated with AI?
What marketing activities are companies currently conducting at the level of whole segments or groups of users? Would the results of these activities improve if the detail level is increased to each user? Where can you extract and how to use such detailed information? How then should the marketing process be changed and automated?
Well, if you can simultaneously increase frequency and improve detail—that would be twice as good 😉
About the Company
OfferFit
Website: offerfit.ai
Last round: $25M, October 24, 2023
Total investments: $39M, rounds: 4