Creating a compelling presentation for investors, tender applications, commercial offers, or even addressing employees can be quite challenging. However, there are general principles of persuasive argumentation that can be taught to an AI. This approach leads to the development of a highly anticipated platform like the one described.
Project Essence
I wrote about this startup in July when it raised 17.2 million pounds sterling (approximately 21.66 million US dollars) in investments. Nevertheless, I couldn’t resist writing about it again – because now it has raised new investments of 39.5 million dollars, thereby increasing the total investment to 65.3 million.
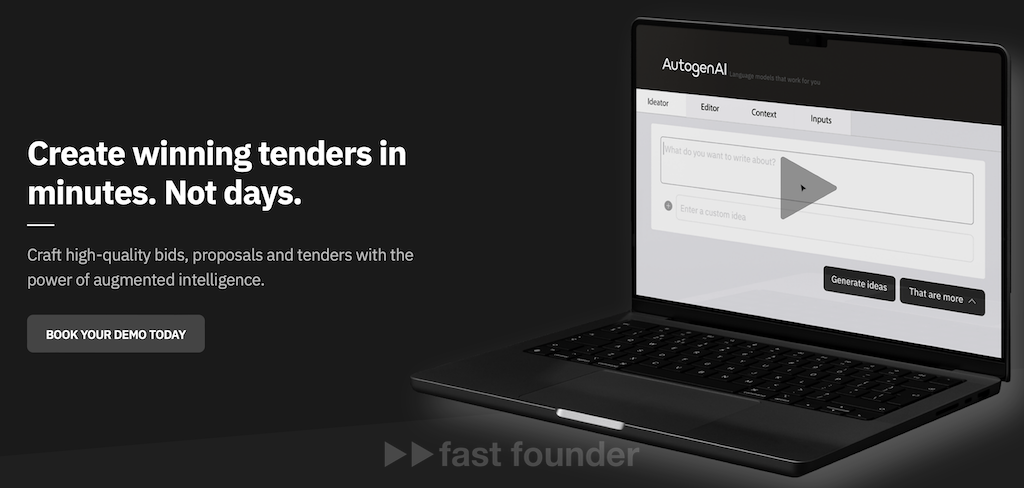
AutogenAI is a platform that allows for the quick and simple creation of compelling applications for participation in tenders, competitions for equipment supply or service provision, and other sales events that need to be won to make money.
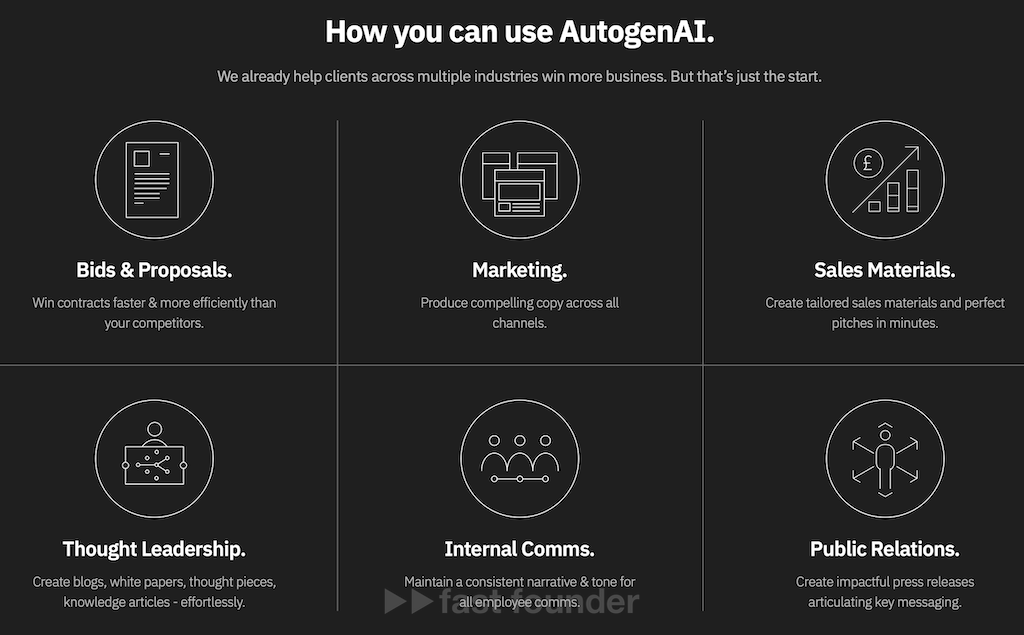
However, the startup’s story began with tender applications. Now, AutogenAI can also be used to create marketing materials, sales proposals for clients, press releases, corporate communications, website and blog articles, and even to write letters to investors describing one’s startup 😉
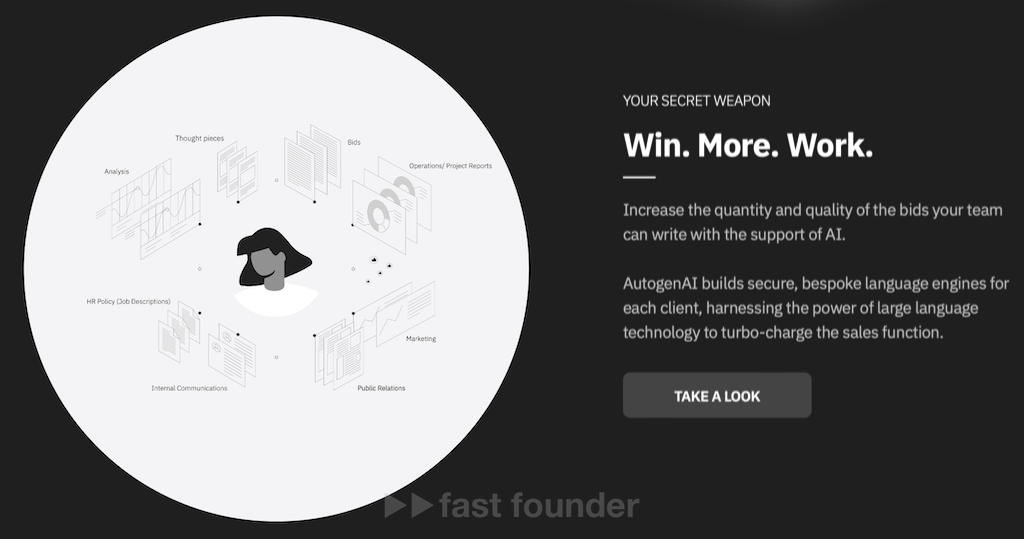
An AI engine, specially trained to create convincing sales texts, powers the platform.
Additionally, the platform can be connected to a company’s documents, including descriptions of the company, its products, past presentations, commercial offers, and other sources of information. The AI engine will then use these sources to extract specific facts about the company and its products and adopt the writing style characteristic of that company.
The general workflow of the platform is as follows.
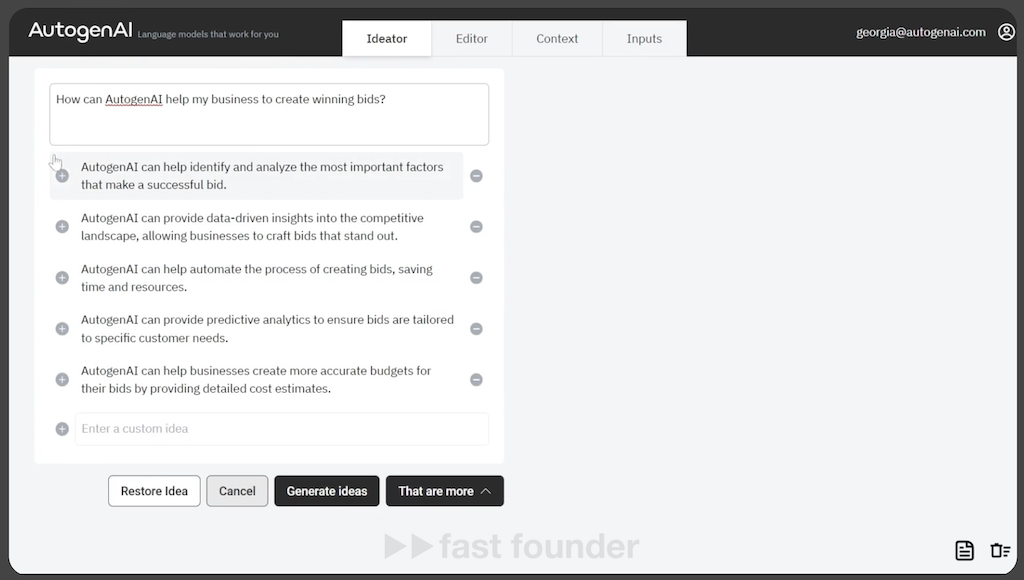
First, you need to set the main theme of the document – for example, “How AutogenAI will help your business sell more.”
Then, the AI engine will present a list of theses – briefly formulated arguments that can be used to substantiate this message. If any of the generated arguments seem unconvincing to the user, they can be removed from the list. Conversely, the user can manually add their argument that the AI engine somehow didn’t use.
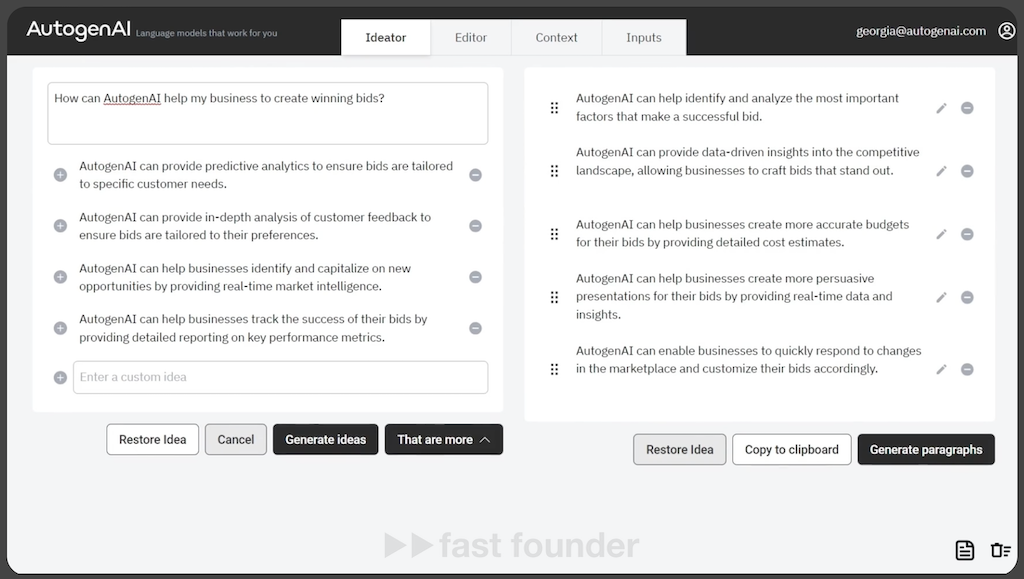
Clicking on a top-level argument, the user gets another list of theses, further elaborating the essence of this argument. Some of these theses can also be deleted, while the remaining ones can be reordered in a more convincing manner.
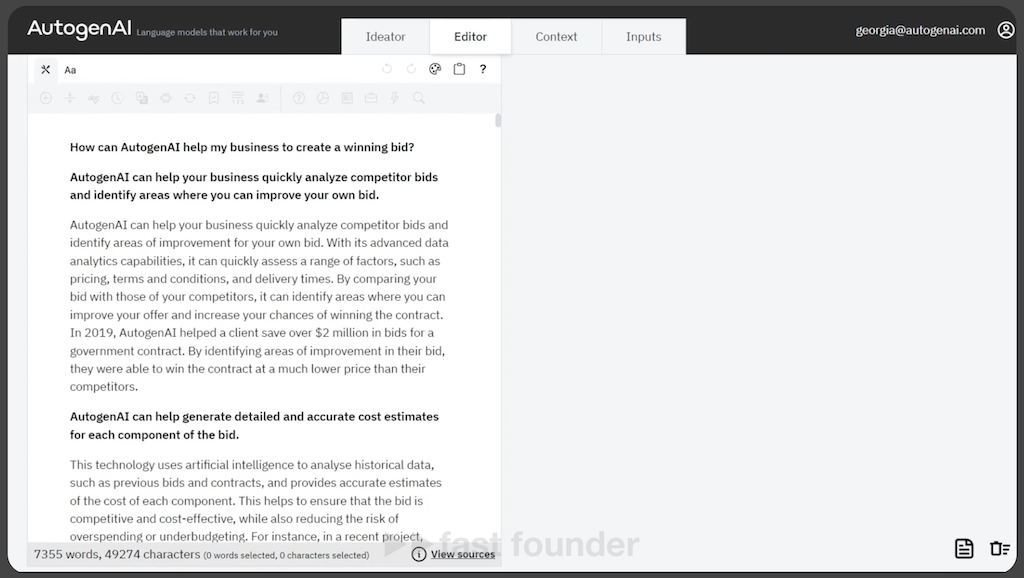
Once the thesis ‘skeleton’ of the document is ready, just press a button – and the AI engine will generate coherent text, smoothly, detailedly, and convincingly unraveling the argumentation.
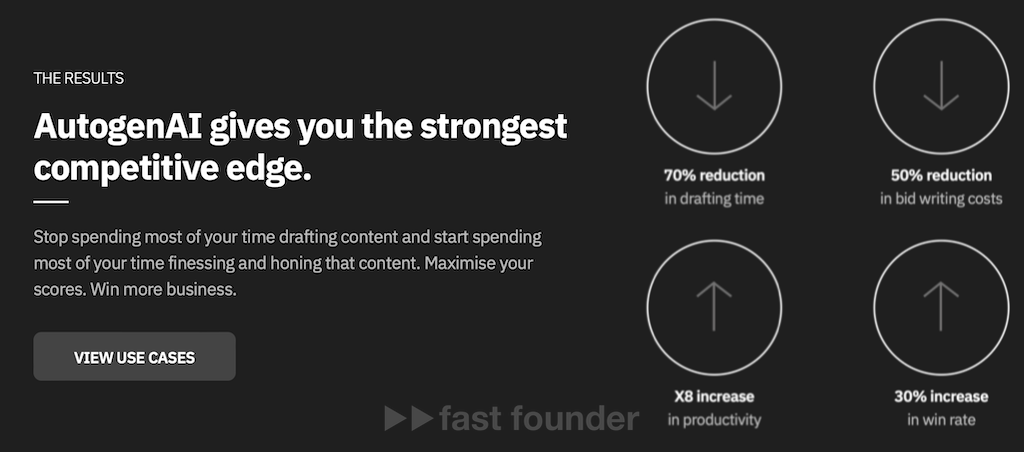
The startup claims that its clients save 70% of the time it takes to create sales documents. But at the same time, they increase the chances of successfully concluding a deal by 30% after sending a document prepared with the platform’s help.
AutogenAI was founded in the United Kingdom but is now actively expanding into the US and Australian markets.
What’s Interesting
Most dreams about AI boil down to the desire to have one magic button – pressing which would yield the perfect result.
However, this usually doesn’t work out, leading to the practice of ‘prompt engineering’ – where users twist and turn different formulations of requests to ChatGPT, trying to get the most satisfying response again by pressing just one button.
If the user is not satisfied with the result, they can repeatedly press the magic button, forcing the AI engine to regenerate the response completely in an unknown manner to them until they get something that more or less satisfies them. Or until they get tired 😉
AutogenAI, as we can see, takes a different approach:
The user first presses buttons to get the ‘skeleton’ of the proposed response, which they can edit manually. Only after that do they press the button to get the final result – which will be composed in a way understandable to them and according to the argumentation scheme they approved. This might seem like a minor technical detail. But in reality, it is an important conceptual point that can change the general principle and interfaces of human-AI interaction.
We can abandon the dream of one magic button – replacing it with a method of successive approximations. First, you should ask the AI to present a general scheme or options of possible schemes of action for the required request. Then choose and/or edit this scheme. And only then ask the AI to deliver the final result – which in this case will be much more predictable and in line with what the user would like to receive.
Ironically, in many cases, such an approach will allow users to get the most satisfying result much faster than through multiple guesses of what and how to ask the AI engine to produce the desired outcome.
Another non-obvious but important point is that the founder of today’s AutogenAI has long been involved in writing tender bids for government contracts.
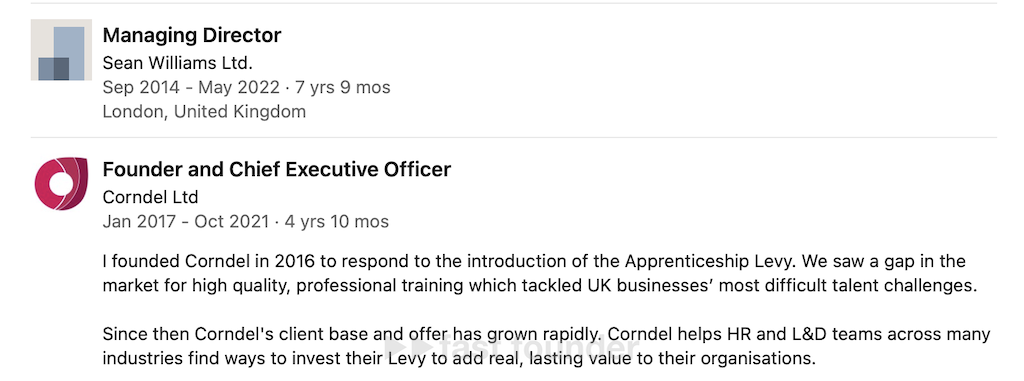
Within the framework of traditional ideology, after gaining such experience, he would have been destined to go into training or consulting – to pass on his accumulated experience to others for a fee. Which, judging by his resume on LinkedIn, he actually did for several years.
However, he eventually stopped teaching people directly – and decided to pass his experience on to AI so that it, in turn, could help people. And, as we see, this turned out to be a much more scalable and profitable way of transferring experience than traditional training and consulting 😉
Where to Head
Next Accordingly, the first direction for potential movement – for those who already consider themselves experts in a particular field and have thought about launching their courses or have already launched them.
Maybe forget about those courses? 😉 A much better option is to teach an AI engine your expertise to create a platform based on it, which can be sold in a much more scalable way.
The second direction for potential movement is to consider in which area of AI application the same method of successive approximations, as used by AutogenAI, can be employed so users can more quickly get predictable and desired results.
What is this area? How is AI already being used there? What intermediate steps should be added to this process to ensure the necessary level of variability and predictability?
And the third direction for those who don’t want to rack their brains over general problems – creating a counterpart of today’s AutogenAI for other languages or specific applications.
In principle, each of the applications listed by AutogenAI – corporate communications, presentations for startups, press releases, etc. – can become a separate product. And the quality of such a product might even be higher than that of the universal AutogenAI, due to better consideration of the specifics of a particular subject area – specific algorithms for generating theses, using additional specific sources of information, training on specially selected data sets, and other fine-tuning.
In which area could such platforms be more in demand? What specifics should be taken into account? What specific datasets can be used to train such an AI engine? What additional sources of information can be used for this?