- Most businesses still operate on the principle of “There are many of you, but only one of me” 😉. And not out of malice, but because there isn’t enough time, effort, patience, and money for each customer. This also applies to digital products ☹️.
- However, AI has made this problem solvable! After all, it can meticulously analyze the behavior of all users and suggest options for how to better activate and retain each of them. And not just suggest—AI can do it itself.
- Therefore, it’s urgent to use such a platform for your own product. Or to create its equivalent to sell to creators of other products 😉.
Project Essence
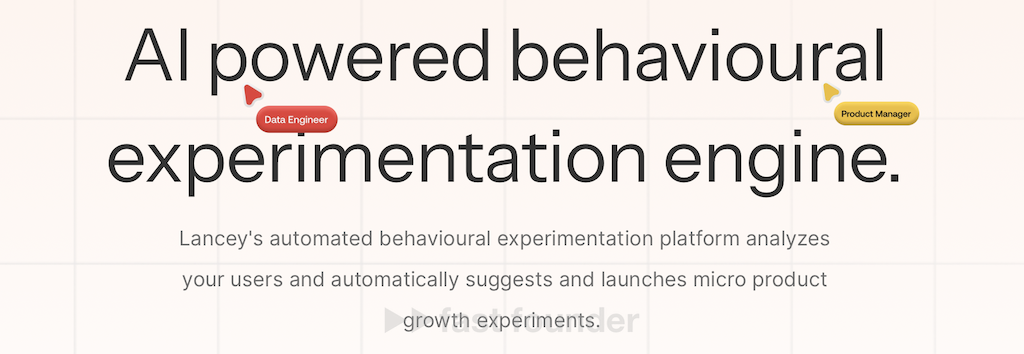
Lancey is a platform for product developers that utilizes AI to conduct “micro-experiments” automatically.

A micro-experiment is an experiment aimed at a small group of product users, selected not only by characteristics but also by previous behavior. This sets micro-experiments apart from traditional product experiments, which are usually conducted for all or large segments of product users—for example, all subscribers to a specific tariff plan.
Why are such micro-experiments necessary? Because all users are different, they behave differently, and they use the product in different ways. As a result, it’s impossible to find one universal way to increase the activity, engagement, or retention of all product users at once.
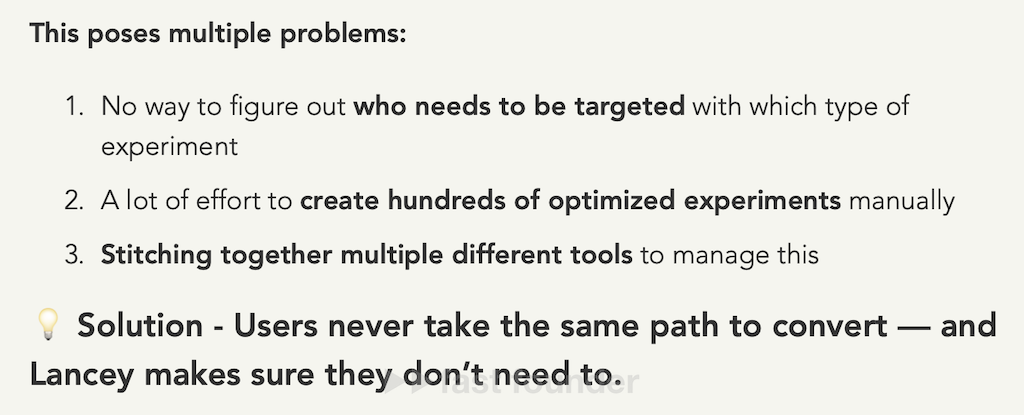
On the other hand, manually analyzing the behavior of all users, dividing them into small groups, conducting experiments for each group, and summarizing the results of these experiments is too cumbersome. Therefore, Lancey decided to simplify this task.
First, all available sources of information about user behavior need to be connected to the Lancey platform—not only within the product but also around it. This information can be taken from Segment, Snowflake, MailChimp, the product payment system, and other sources. Ready-made integration modules are already available on the Lancey platform for the most popular ones.
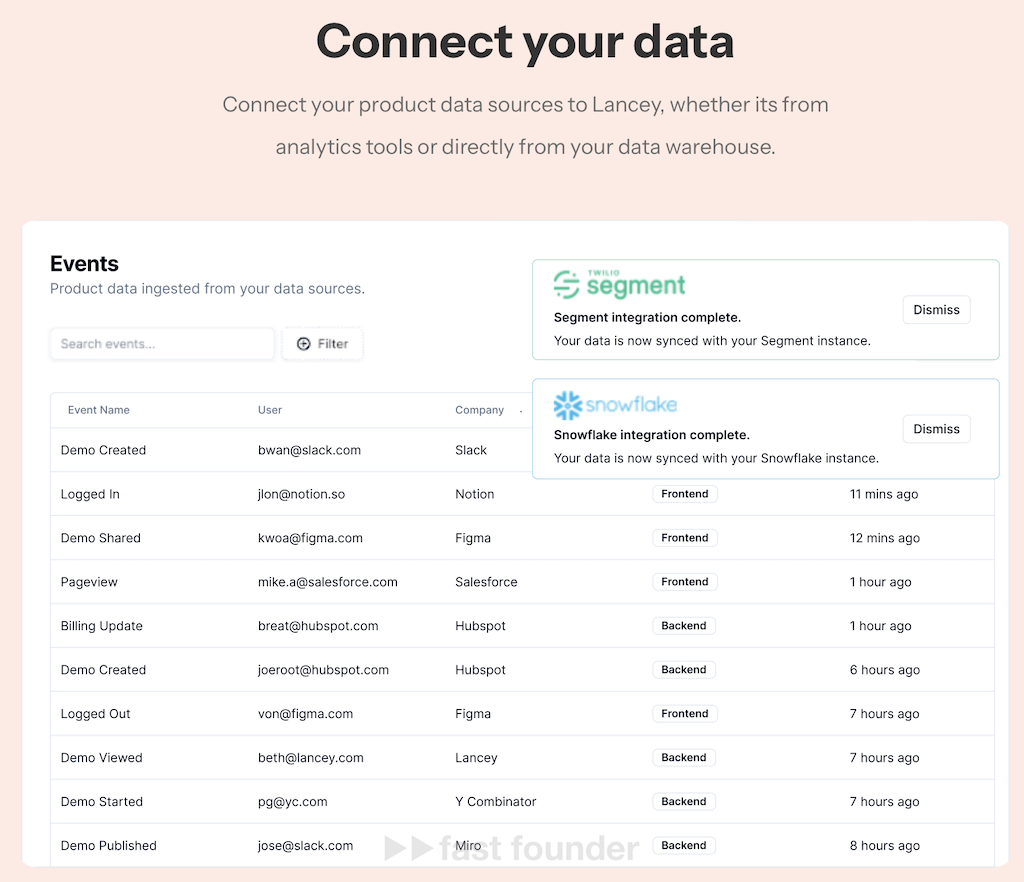
Next, you need to set the goal of the experiments—what do we want? To increase the frequency of product usage, engagement, retain users, or something else.
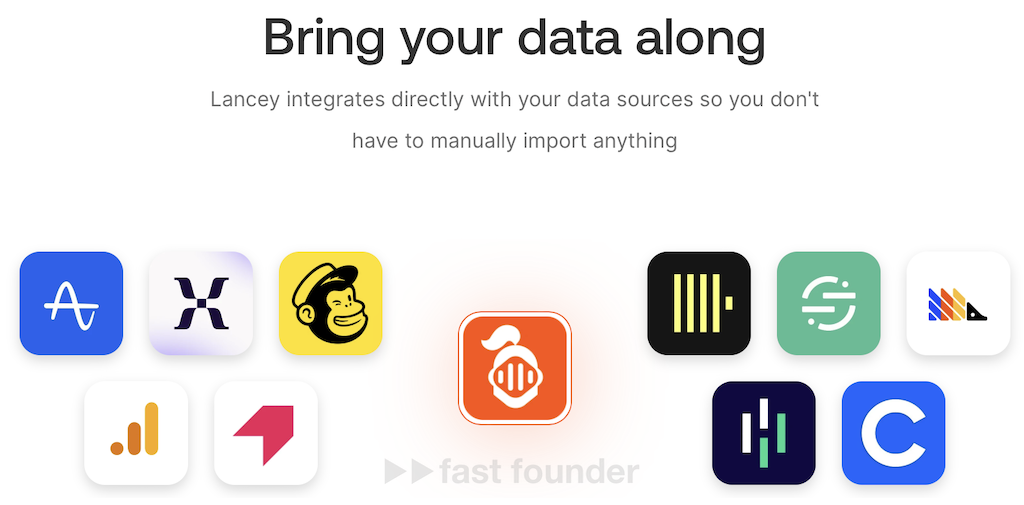
After that, Lancey’s AI engine will automatically identify user characteristics and behavior patterns that most strongly influence the metrics of this goal.
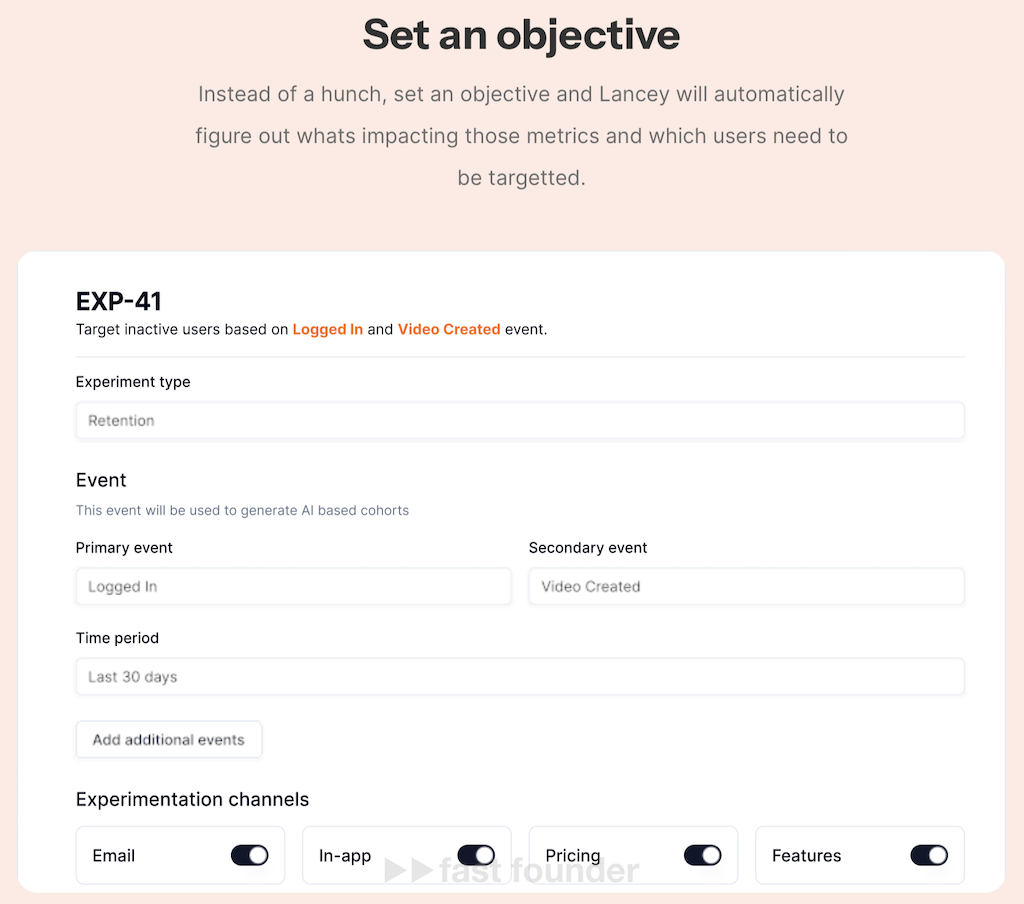
At the next stage, the AI engine will divide all users into different groups depending on the values of the identified characteristics and behavior patterns.
Now the AI engine can compile a list of experiments that can be conducted with a small sample from each group of users to assess their impact on the results of the target metric—by comparing the results of the experiment obtained from the sample of users with the results of the control group (users from the same group not affected by the experiment).
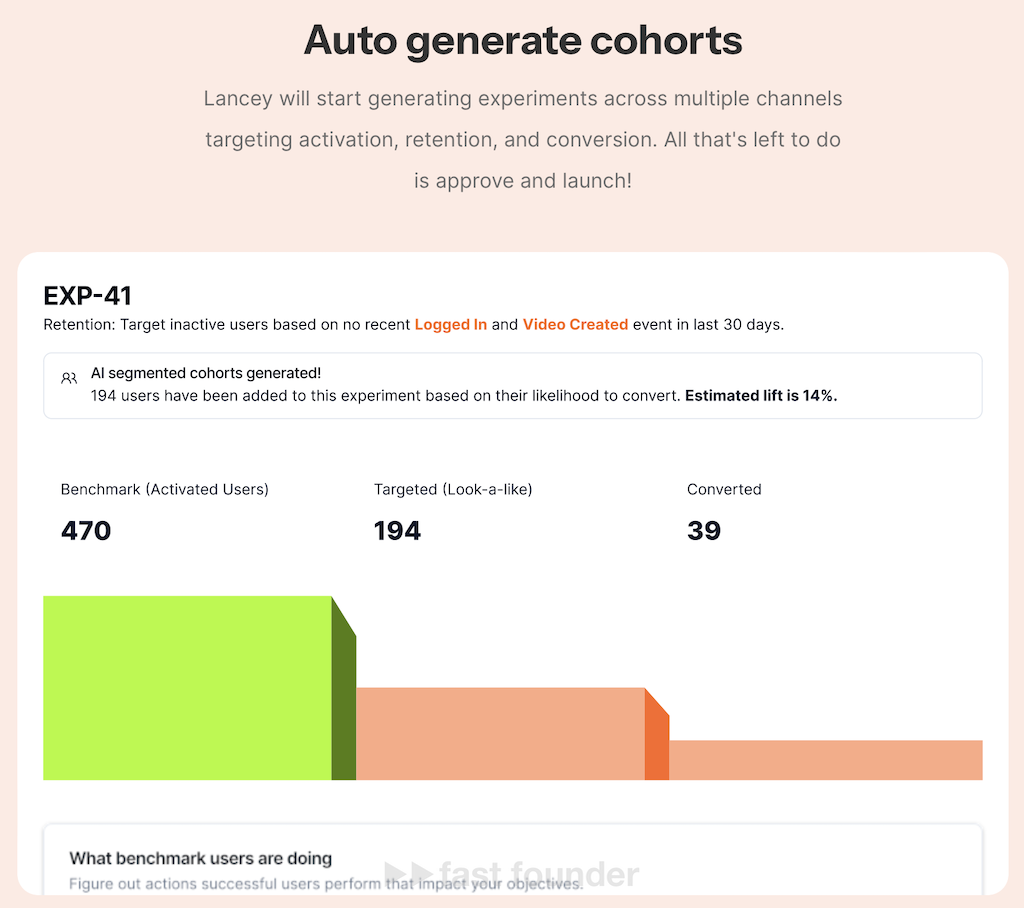
To conduct an experiment, you need to program the essence of the experiment as a separate procedure—for example, showing a banner with a specific call to action. Then the platform can call this procedure to conduct the experiment on the required sample of users.
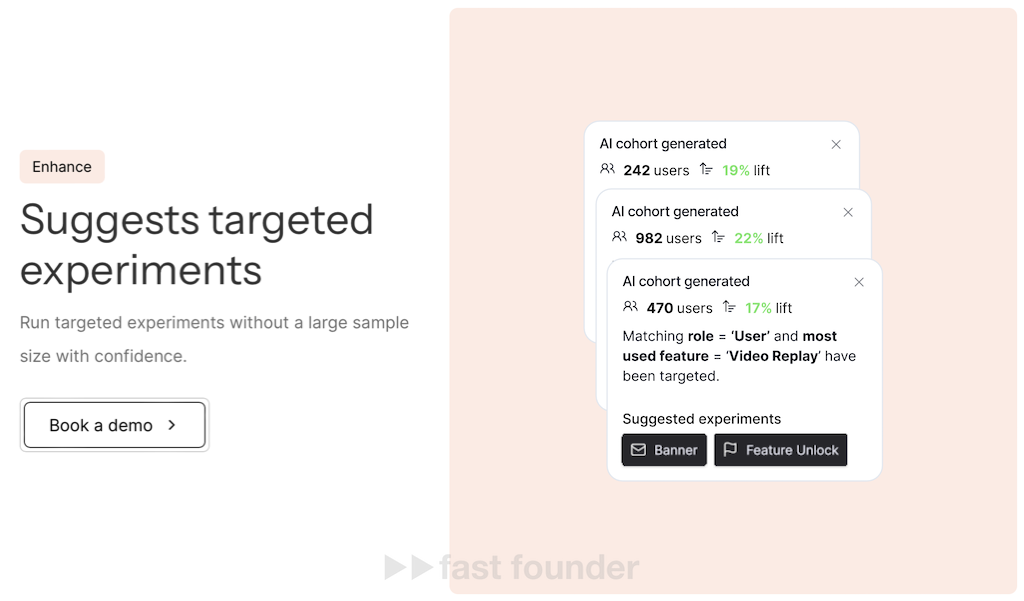
As a result of conducting such individual experiments, a library of different procedures for “spurring” users begins to accumulate. This means that now Lancey can be switched to autopilot mode.
In this mode, the AI engine of the platform can automatically and regularly analyze user behavior, divide them into groups, come up with and conduct experiments using the available library of procedures, summarize their results—and roll out to the entire group of users a set of procedures that successfully stimulate them on the way to the set goal.
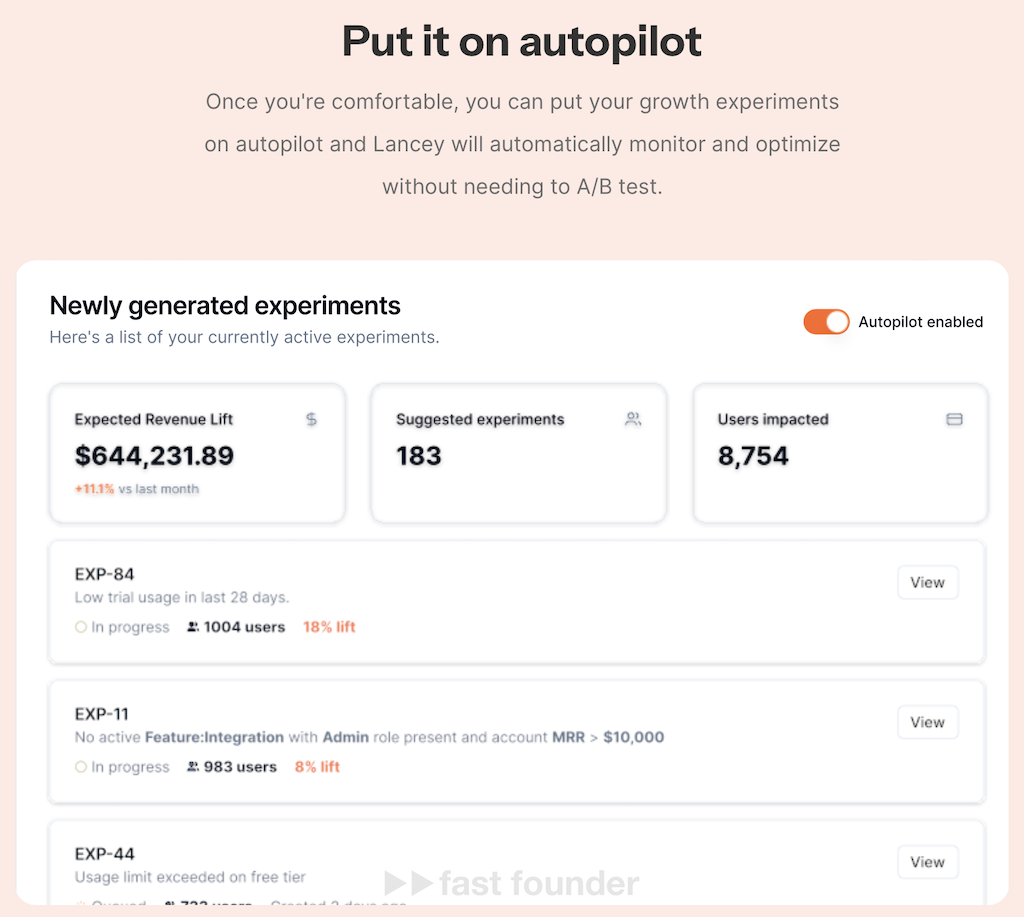
Lancey went through Y Combinator in the summer of 2022, receiving the allocated $500,000 investment. However, they only launched the beta version of the described platform a few days ago.
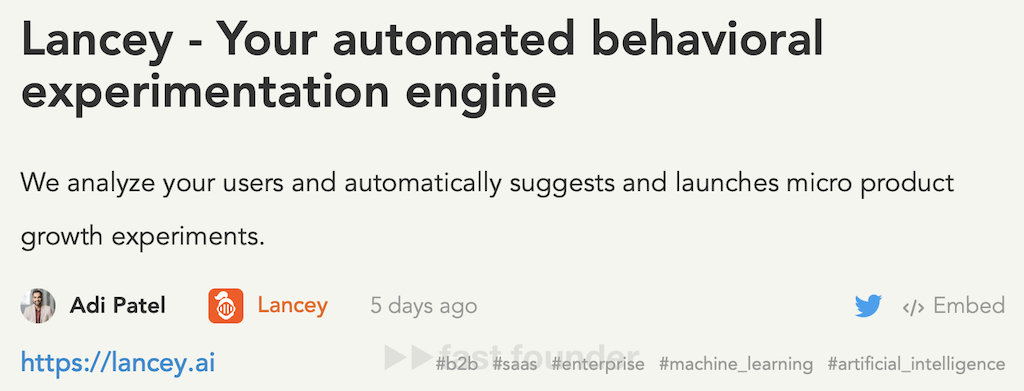
What’s Interesting
Most businesses still operate on the principle of “There are many of you, and I’m alone” 😉. And it’s not out of malice but because they lack the strength, time, patience, and money to deal with each individual customer ☹️.
The same is happening with digital products. Delving into how individual users behave, what influences their behavior, and how this behavior affects product usage results is too meticulous a task for product developers who lack the strength, time, patience, and money. It’s easier and quicker to make decisions with a “one-size-fits-all” approach, hoping that it will mainly lead to the desired results.
However, developers lose part of the potential benefit from the “long tail” of other users because they don’t count on them when making such decisions. The wider the product’s functionality, the longer this tail will be 😉. Since different users will use different product features for different purposes—and adapting to all of them will be even more problematic.
The situation worsens further because users’ stratification based on different behavior patterns can even occur in simple products. In one of the recent reviews, I gave an example that even FastFounder readers read it very differently—some on the website, some on Telegram, some every morning for one new review, some for seven reviews at once weekly after the newsletter, or on Sundays, some for half a day once every few months.
As a result, to retain subscribers, you can’t try to encourage everyone to follow one behavior pattern—because it will negatively impact everyone else.
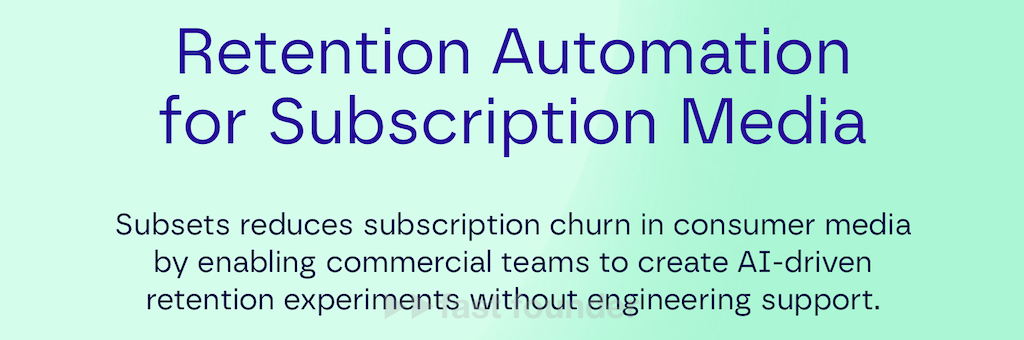
That review was dedicated to the Subsets startup, which made a platform to reduce churn of subscribers from subscription digital publications.
Its feature lies precisely in the fact that their platform’s AI analyzes reader behavior to identify different behavior patterns and helps find different sets of actions to retain subscribers with different patterns.
Today’s Lancey conceptually resembles Subsets but, unlike it, can work with different types of products, not just internet publications.
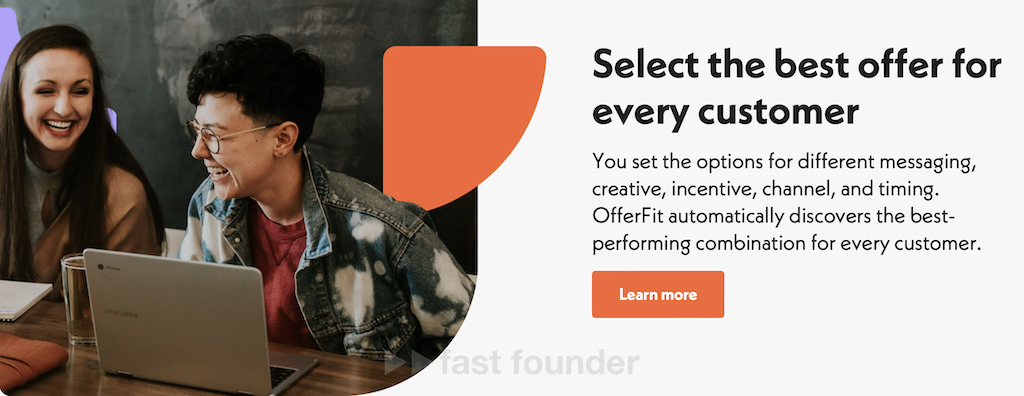
A similar scheme is employed by the OfferFit startup, which I wrote about in November. They created a platform for sending offers to buyers, which also takes into account their personal characteristics—what they buy more often, what they bite on more, and even when they prefer to read emails or text messages. Thus, each buyer can receive an individual offer on an individual topic, formulated individually, and sent at an individual time.
In summary, the concepts of Lancey, Subsets, and OfferFit can be called “scalable personalization”—when we get the opportunity to tailor to each user but do it on a large scale without spending extra effort, time, and money on such customization.
Dividing users into small groups is a particular (or general?) case of such individual adjustment because although each person is unique, some of them are similar in some ways to others 😉
It’s clear that such “scalable personalization” became possible only thanks to AI technologies, which can engage in meticulous user analysis and meticulous adjustment to them automatically.
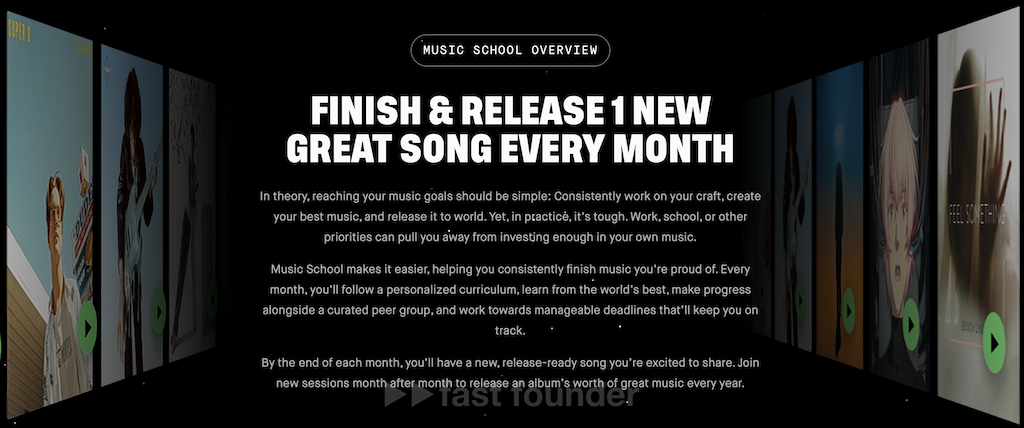
This concept works not only for subscription services or stores. Recently, I wrote about a fairly large startup, Studio, which has already raised $60 million in investments and created a website with online courses on various topics, including music.
So, they recently launched the “music school of the future,” promising that each student of this school will be able to release one worthy song every month! Its feature also lies in the fact that they created an AI instructor that selects an individual set of lessons for each student and provides individual assistance in creating their song.
Without an AI instructor, such a promise couldn’t work because it would be too expensive to have live teachers who can constantly deal with each student individually. By the way, this startup’s students also form small groups of up to 20 people based on the similarity of their initial level and goals.
Where to Run
The direction to head towards is “scalable personalization” 😉. Because the problem faced by product creators is clear, and the method of solving this problem using AI has only recently emerged.
The first path is to use AI for meticulous analysis and customization of one’s own product for different types of users to improve their activation, engagement, and retention metrics.
The second path is to create platforms that allow other product developers to do the same.
Even looking at the examples of startups mentioned today, we see that this concept can be applied in many places and in various ways.
Subscription services, stores, and educational services are already obvious options. However, we can still focus on them—by starting with creating an analog of one of these platforms. After all, these markets are large enough to accommodate many players.
But the interesting question is—on what other markets and for what purposes can the same concept of scalable personalization be applied? Where else do users, clients, or customers demonstrate different behavior patterns en masse while using the same products? How can one adapt to such diverse patterns, increasing the revenue for the creators of these products?
About the Company
Lancey
Website: lancey.ai
Latest Round: $500K, 08/01/2022
Total Investments: $500K, Rounds: 1